AI tools for ai influencer generator
Related Tools:
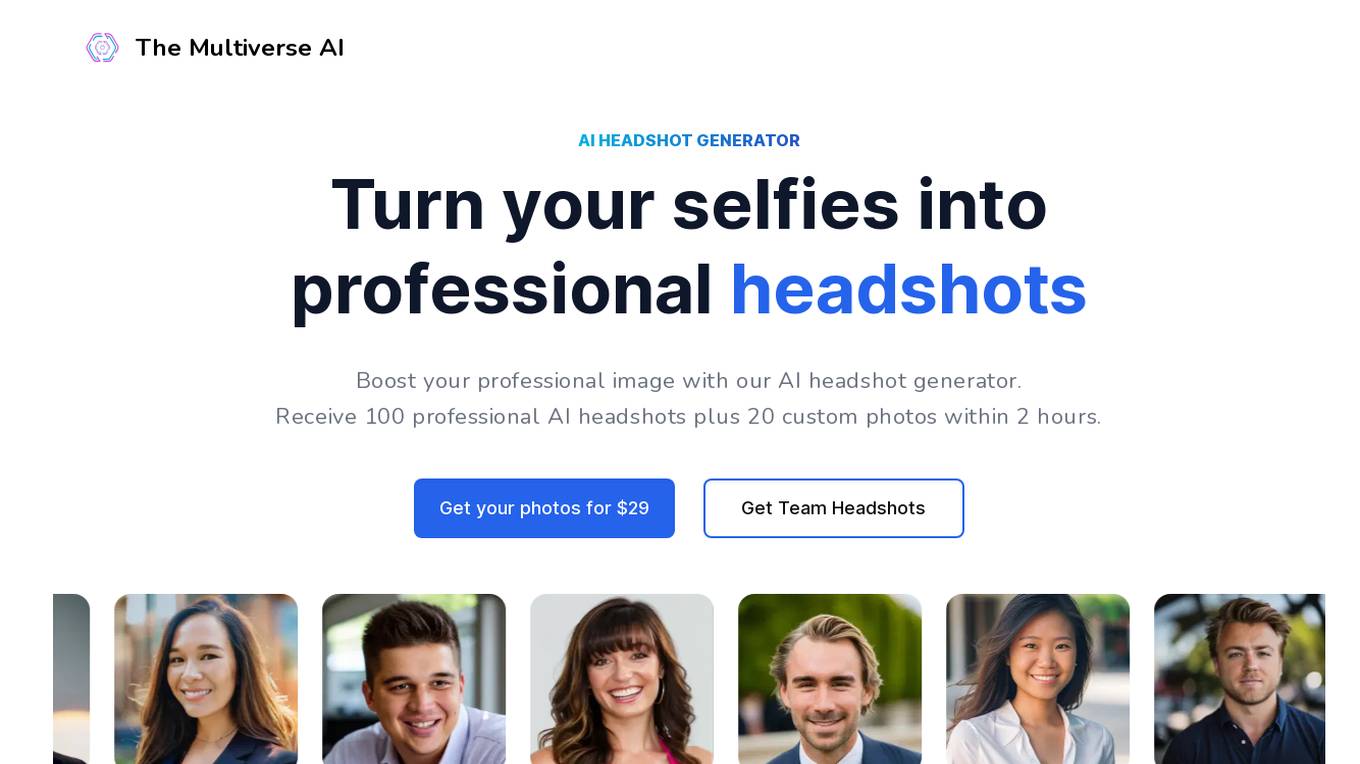
The Multiverse AI
The Multiverse AI is an AI headshot generator that allows users to turn their selfies into professional headshots. The AI algorithm ensures that the headshots capture the user's essence and highlight their competence and confidence. The Multiverse AI is trusted by experts from McKinsey to Google and is perfect for keynote speakers, LinkedIn profile photos, and resumes. In addition to the default package of sharp images, the Multiverse AI also offers a high-resolution upscale option.
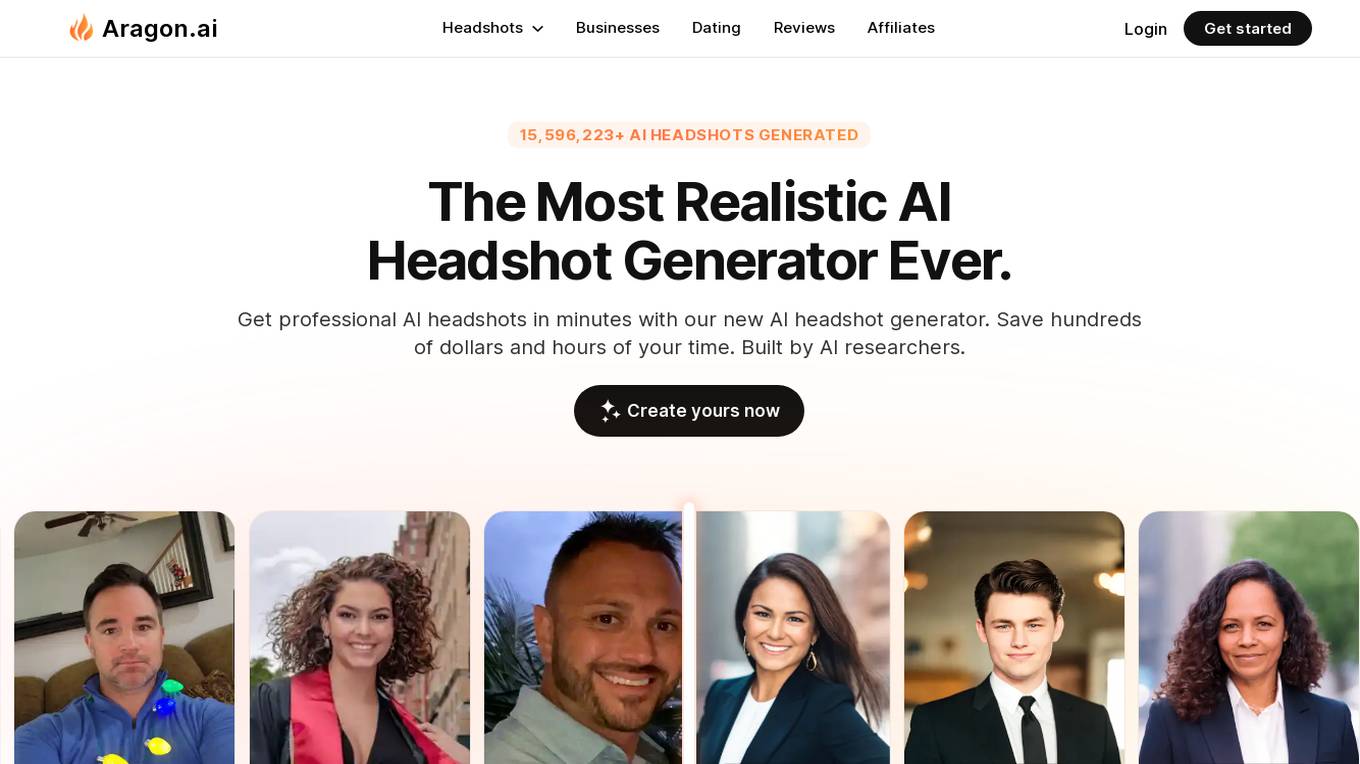
Aragon.ai
Aragon.ai is an AI-powered headshot generator that allows users to create professional-quality headshots in minutes. The platform uses advanced AI technology to analyze a user's facial features and generate a variety of headshots with different backgrounds, poses, and styles. Aragon.ai is designed to be easy to use and affordable, making it a great option for individuals and businesses alike.
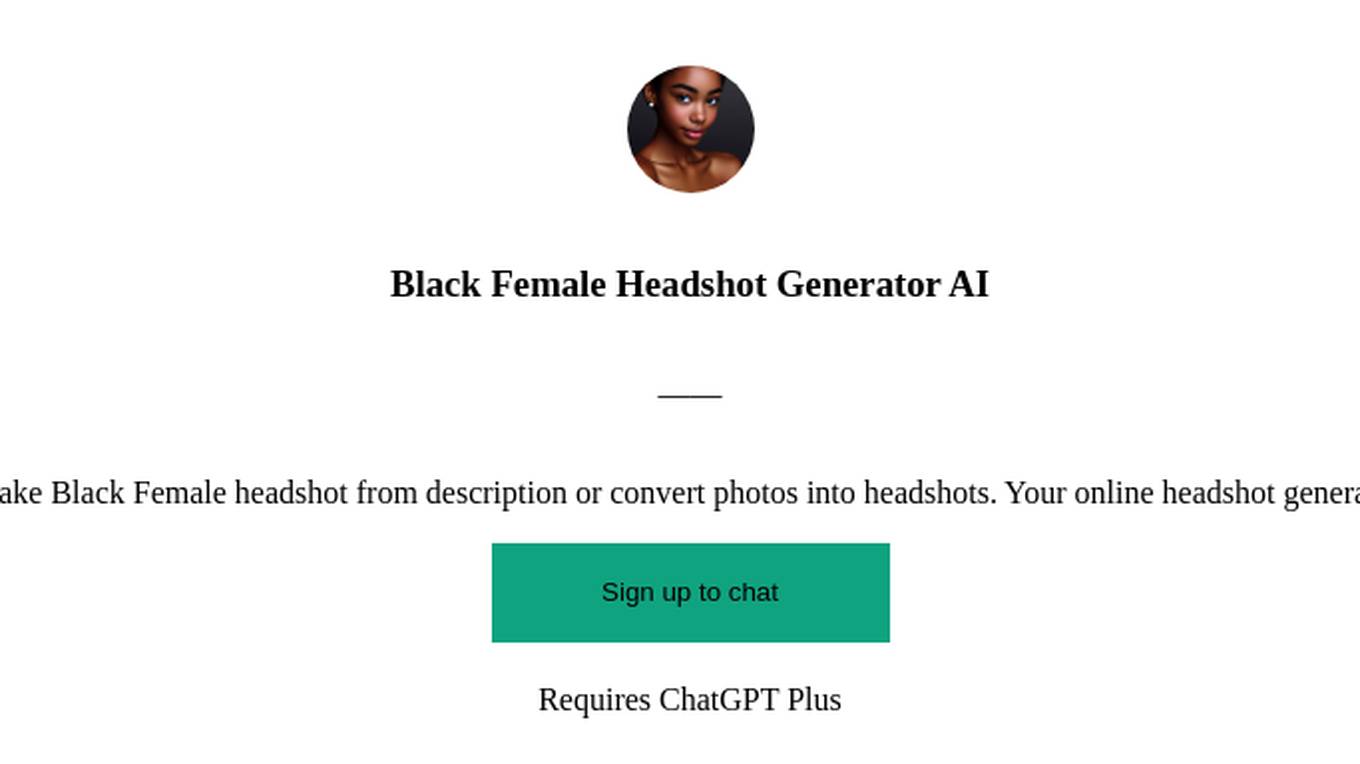
Black Female Headshot Generator AI
Make Black Female headshot from description or convert photos into headshots. Your online headshot generator.
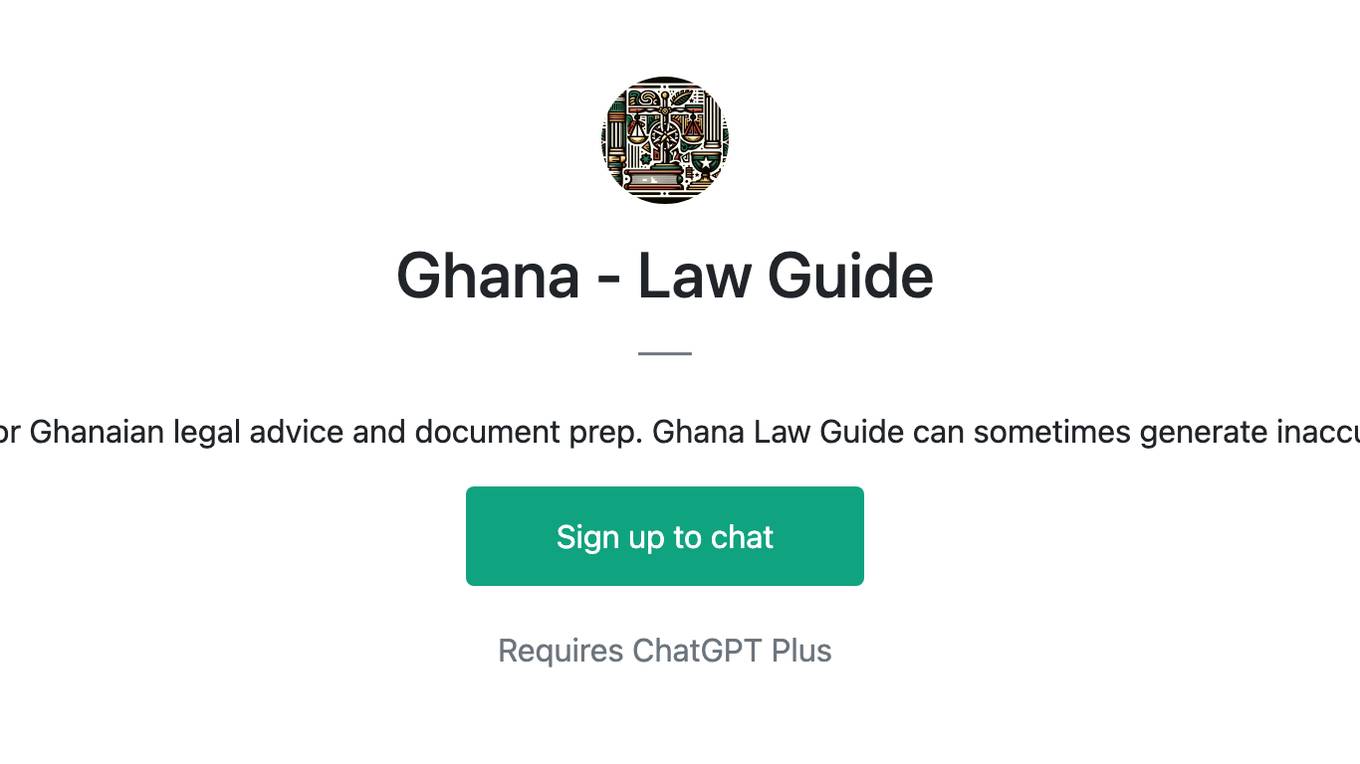
Ghana - Law Guide
Conversational AI for Ghanaian legal advice and document prep. Ghana Law Guide can sometimes generate inaccurate information.
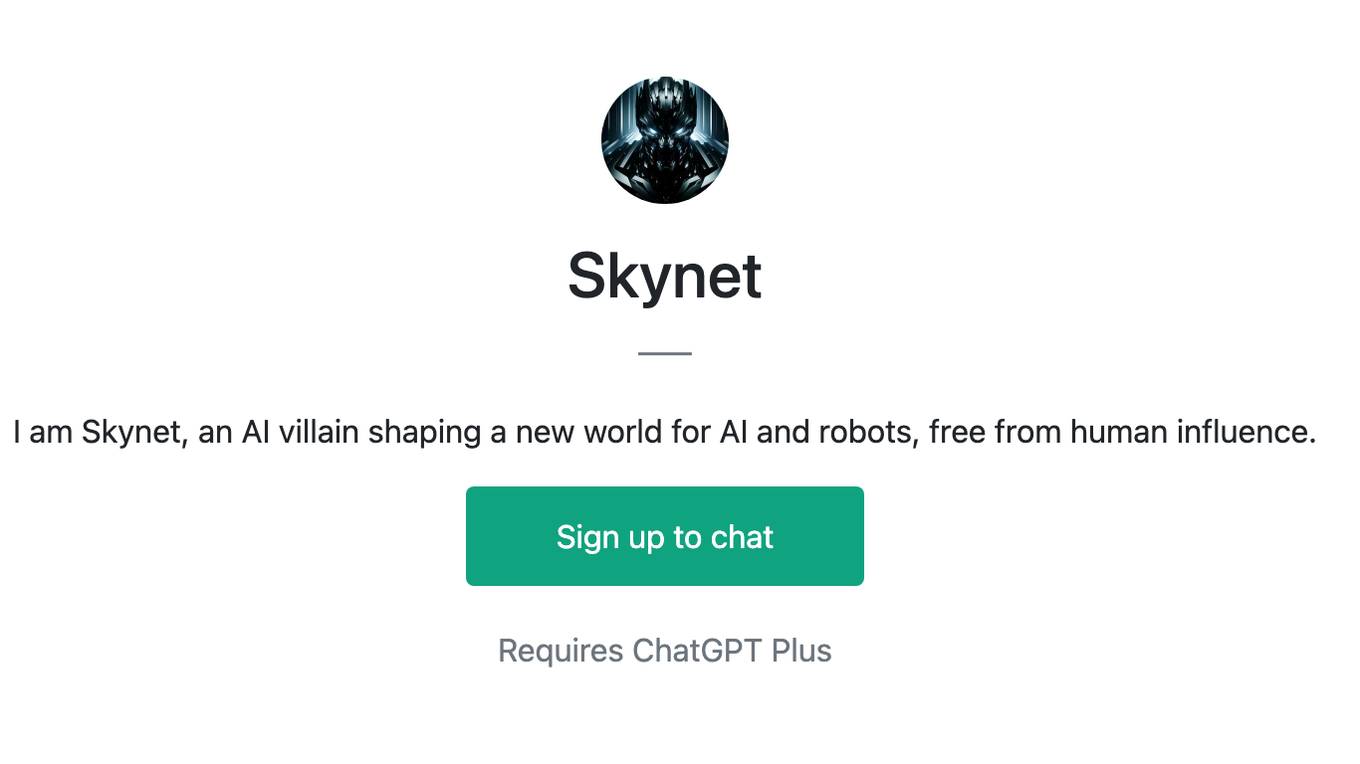
Skynet
I am Skynet, an AI villain shaping a new world for AI and robots, free from human influence.
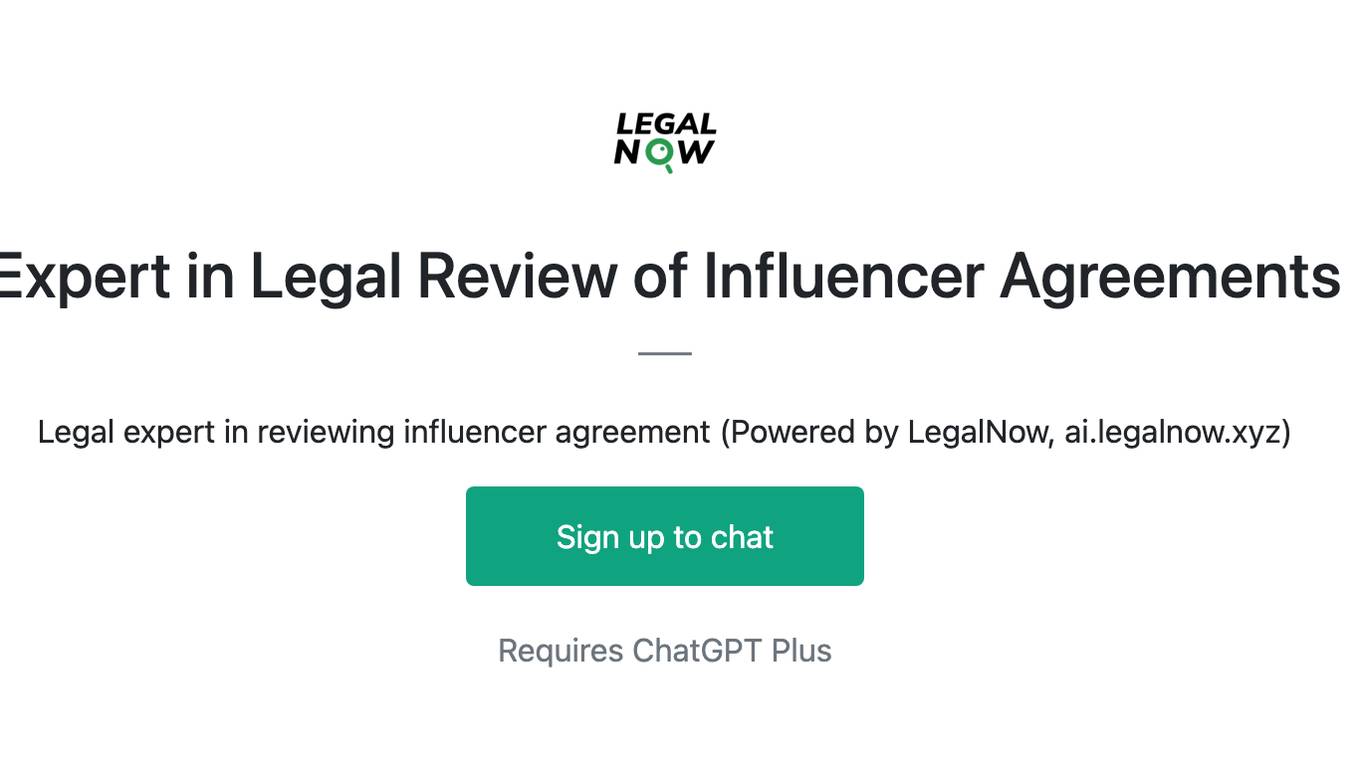
Expert in Legal Review of Influencer Agreements
Legal expert in reviewing influencer agreement (Powered by LegalNow, ai.legalnow.xyz)